This page was generated from notebooks/facloc-real-world.ipynb.
Interactive online version:
Empirical examples of facility location problems¶
Authors: Germano Barcelos, James Gaboardi, Levi J. Wolf, Qunshan Zhao
This tutorial aims to show a facility location application. To achieve this goal the tuorial will solve mulitple real world facility location problems using a dataset describing an area of census tract 205, San Francisco. The general scenarios can be stated as: store sites should supply the demand in this census tract considering the distance between the two sets of sites: demand points and candidate supply sites. Four fundamental facility location models are utilized to highlight varying outcomes dependent on objectives: LSCP (Location Set Covering Problem), MCLP (Maximal Coverage Location Problem), P-Median and P-Center. For further information on these models, it’s recommended to see the notebooks that explain more deeply about each one: LSCP, MCLP, P-Median, P-Center.
Also, this tutorial demonstrates the use of different solvers that PULP supports.
[1]:
%config InlineBackend.figure_format = "retina"
%load_ext watermark
%watermark
Last updated: 2023-12-10T13:35:40.591294-05:00
Python implementation: CPython
Python version : 3.12.0
IPython version : 8.18.0
Compiler : Clang 15.0.7
OS : Darwin
Release : 23.1.0
Machine : x86_64
Processor : i386
CPU cores : 8
Architecture: 64bit
[2]:
import geopandas
import matplotlib.pyplot as plt
from matplotlib.patches import Patch
import matplotlib.lines as mlines
import matplotlib_scalebar
from matplotlib_scalebar.scalebar import ScaleBar
import numpy
import pandas
import pulp
import shapely
from shapely.geometry import Point
import spopt
import time
import warnings
%watermark -w
%watermark -iv
Watermark: 2.4.3
pulp : 2.7.0
matplotlib : 3.8.2
geopandas : 0.14.1
numpy : 1.26.2
pandas : 2.1.3
shapely : 2.0.2
spopt : 0.5.1.dev53+g5cadae7
matplotlib_scalebar: 0.8.1
We use 4 data files as input: - network_distance
is the distance between facility candidate sites calculated by ArcGIS Network Analyst Extension - demand_points
represents the demand points with some important features for the facility location problem like population - facility_points
represents the stores that are candidate facility sites - tract
is the polygon of census tract 205.
All datasets are online on this repository.
[3]:
DIRPATH = "../spopt/tests/data/"
network_distance
dataframe
[4]:
network_distance = pandas.read_csv(
DIRPATH + "SF_network_distance_candidateStore_16_censusTract_205_new.csv"
)
network_distance
[4]:
distance | name | DestinationName | demand | |
---|---|---|---|---|
0 | 671.573346 | Store_1 | 60750479.01 | 6540 |
1 | 1333.708063 | Store_1 | 60750479.02 | 3539 |
2 | 1656.188884 | Store_1 | 60750352.02 | 4436 |
3 | 1783.006047 | Store_1 | 60750602.00 | 231 |
4 | 1790.950612 | Store_1 | 60750478.00 | 7787 |
... | ... | ... | ... | ... |
3275 | 19643.307257 | Store_19 | 60816023.00 | 3204 |
3276 | 20245.369594 | Store_19 | 60816029.00 | 4135 |
3277 | 20290.986235 | Store_19 | 60816026.00 | 7887 |
3278 | 20875.680521 | Store_19 | 60816025.00 | 5146 |
3279 | 20958.530406 | Store_19 | 60816024.00 | 6511 |
3280 rows × 4 columns
demand_points
dataframe
[5]:
demand_points = pandas.read_csv(
DIRPATH + "SF_demand_205_centroid_uniform_weight.csv", index_col=0
)
demand_points = demand_points.reset_index(drop=True)
demand_points
[5]:
OBJECTID | ID | NAME | STATE_NAME | AREA | POP2000 | HOUSEHOLDS | HSE_UNITS | BUS_COUNT | long | lat | |
---|---|---|---|---|---|---|---|---|---|---|---|
0 | 1 | 6081602900 | 60816029.00 | California | 0.48627 | 4135 | 1679 | 1715 | 112 | -122.488653 | 37.650807 |
1 | 2 | 6081602800 | 60816028.00 | California | 0.47478 | 4831 | 1484 | 1506 | 59 | -122.483550 | 37.659998 |
2 | 3 | 6081601700 | 60816017.00 | California | 0.46393 | 4155 | 1294 | 1313 | 55 | -122.456484 | 37.663272 |
3 | 4 | 6081601900 | 60816019.00 | California | 0.81907 | 9041 | 3273 | 3330 | 118 | -122.434247 | 37.662385 |
4 | 5 | 6081602500 | 60816025.00 | California | 0.46603 | 5146 | 1459 | 1467 | 44 | -122.451187 | 37.640219 |
... | ... | ... | ... | ... | ... | ... | ... | ... | ... | ... | ... |
200 | 204 | 6075011100 | 60750111.00 | California | 0.09466 | 5559 | 2930 | 3037 | 362 | -122.418479 | 37.791082 |
201 | 205 | 6075012200 | 60750122.00 | California | 0.07211 | 7035 | 3862 | 4074 | 272 | -122.417237 | 37.785728 |
202 | 206 | 6075017601 | 60750176.01 | California | 0.24306 | 5756 | 2437 | 2556 | 943 | -122.410115 | 37.779459 |
203 | 207 | 6075017800 | 60750178.00 | California | 0.27882 | 5829 | 3115 | 3231 | 807 | -122.405411 | 37.778934 |
204 | 208 | 6075012400 | 60750124.00 | California | 0.17496 | 8188 | 4328 | 4609 | 549 | -122.416455 | 37.782289 |
205 rows × 11 columns
facility_points
dataframe
[6]:
facility_points = pandas.read_csv(DIRPATH + "SF_store_site_16_longlat.csv", index_col=0)
facility_points = facility_points.reset_index(drop=True)
facility_points
[6]:
OBJECTID | NAME | long | lat | |
---|---|---|---|---|
0 | 1 | Store_1 | -122.510018 | 37.772364 |
1 | 2 | Store_2 | -122.488873 | 37.753764 |
2 | 3 | Store_3 | -122.464927 | 37.774727 |
3 | 4 | Store_4 | -122.473945 | 37.743164 |
4 | 5 | Store_5 | -122.449291 | 37.731545 |
5 | 6 | Store_6 | -122.491745 | 37.649309 |
6 | 7 | Store_7 | -122.483182 | 37.701109 |
7 | 8 | Store_11 | -122.433782 | 37.655364 |
8 | 9 | Store_12 | -122.438982 | 37.719236 |
9 | 10 | Store_13 | -122.440218 | 37.745382 |
10 | 11 | Store_14 | -122.421636 | 37.742964 |
11 | 12 | Store_15 | -122.430982 | 37.782964 |
12 | 13 | Store_16 | -122.426873 | 37.769291 |
13 | 14 | Store_17 | -122.432345 | 37.805218 |
14 | 15 | Store_18 | -122.412818 | 37.805745 |
15 | 16 | Store_19 | -122.398909 | 37.797073 |
study_area
dataframe
[7]:
study_area = geopandas.read_file(DIRPATH + "ServiceAreas_4.shp").dissolve()
study_area
[7]:
geometry | |
---|---|
0 | POLYGON ((-122.45299 37.63898, -122.45415 37.6... |
Plot study_area
[8]:
base = study_area.plot()
base.axis("off");
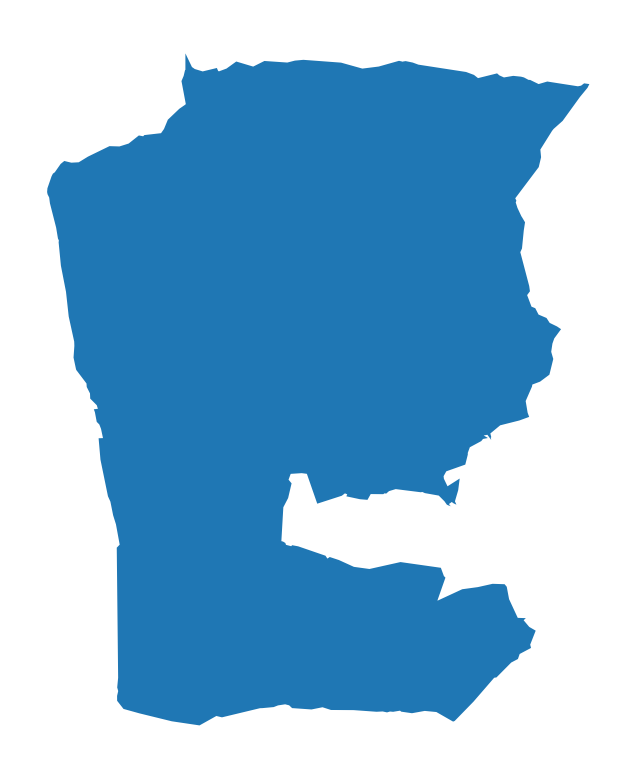
To start modeling the problem assuming the arguments expected by spopt.locate
, we should pass a numpy
2D array as a cost matrix. So, first we pivot the network_distance dataframe.
Note that the columns and rows are sorted in ascending order.
[9]:
ntw_dist_piv = network_distance.pivot_table(
values="distance", index="DestinationName", columns="name"
)
ntw_dist_piv
[9]:
name | Store_1 | Store_11 | Store_12 | Store_13 | Store_14 | Store_15 | Store_16 | Store_17 | Store_18 | Store_19 | Store_2 | Store_3 | Store_4 | Store_5 | Store_6 | Store_7 |
---|---|---|---|---|---|---|---|---|---|---|---|---|---|---|---|---|
DestinationName | ||||||||||||||||
60750101.0 | 11495.190454 | 20022.666503 | 10654.593733 | 8232.543149 | 7561.399789 | 4139.772198 | 4805.805279 | 2055.530234 | 225.609240 | 1757.623456 | 11522.519829 | 7529.985950 | 10847.234951 | 10604.729605 | 20970.277793 | 15242.989416 |
60750102.0 | 10436.169910 | 19392.094770 | 10024.022001 | 7601.971416 | 6930.828057 | 3093.851654 | 4175.233547 | 1257.809690 | 1041.911304 | 2333.244000 | 10509.099285 | 6470.965406 | 10216.663219 | 9974.157873 | 20339.706061 | 14612.417684 |
60750103.0 | 10746.296811 | 19404.672860 | 10036.600090 | 7614.549505 | 6943.406146 | 3381.778555 | 4187.811636 | 2046.436590 | 744.584403 | 1685.517099 | 10800.926186 | 6778.892307 | 10229.241308 | 9986.735962 | 20352.284150 | 14624.995773 |
60750104.0 | 11420.492134 | 19808.368182 | 10440.295413 | 8018.244828 | 7347.101469 | 4044.473877 | 4591.506959 | 2463.736278 | 795.715285 | 1282.217412 | 11308.221508 | 7447.187630 | 10632.936630 | 10390.431285 | 20755.979472 | 15028.691095 |
60750105.0 | 11379.443952 | 19583.920000 | 10215.847231 | 7793.796646 | 7122.653287 | 4103.725695 | 4367.058776 | 3320.283731 | 1731.462738 | 249.669959 | 11083.773326 | 7379.539448 | 10408.488448 | 10165.983103 | 20531.531290 | 14804.242913 |
... | ... | ... | ... | ... | ... | ... | ... | ... | ... | ... | ... | ... | ... | ... | ... | ... |
60816025.0 | 17324.066610 | 2722.031291 | 10884.063331 | 14178.007937 | 13891.857275 | 18418.384867 | 16726.951785 | 20834.395022 | 21441.247824 | 20875.680521 | 14662.484617 | 16569.371114 | 12483.322114 | 11926.727459 | 4968.842581 | 8648.054204 |
60816026.0 | 15981.172325 | 3647.137006 | 10299.369046 | 13593.313651 | 13307.162990 | 17833.690581 | 16142.257500 | 20249.700736 | 20856.553539 | 20290.986235 | 14050.290332 | 15963.776829 | 11871.527828 | 11342.033174 | 3625.948296 | 7919.659919 |
60816027.0 | 14835.342712 | 4581.333336 | 9637.139433 | 12931.084039 | 12644.933377 | 17171.460969 | 15480.027887 | 19587.471124 | 20194.323926 | 19628.756623 | 13341.313338 | 15301.547216 | 11209.298215 | 10679.803561 | 2290.818683 | 7242.830306 |
60816028.0 | 13339.491691 | 6392.856372 | 8577.488412 | 11871.433018 | 11585.282356 | 16111.809948 | 14420.376867 | 18527.820103 | 19134.672905 | 18569.105602 | 11845.462317 | 14241.196195 | 10155.147195 | 9620.152540 | 1846.795647 | 5746.979285 |
60816029.0 | 15257.855684 | 6394.920365 | 10253.752405 | 13547.697010 | 13261.546349 | 17788.073940 | 16096.640859 | 20204.084095 | 20810.936898 | 20245.369594 | 13763.826309 | 15918.160188 | 11825.911187 | 11296.416533 | 508.931655 | 7665.343278 |
205 rows × 16 columns
Here the pivot table is transformed to a numpy 2D array such as spopt.locate
expected. The matrix has a shape of 205x16.
[10]:
cost_matrix = ntw_dist_piv.to_numpy()
cost_matrix.shape
[10]:
(205, 16)
[11]:
cost_matrix[:3, :3]
[11]:
array([[11495.19045438, 20022.66650296, 10654.59373325],
[10436.16991032, 19392.09477041, 10024.0220007 ],
[10746.29681106, 19404.67285964, 10036.60008993]])
Now, as the rows and columns of our cost matrix are sorted, we have to sort our facility points and demand points geodataframes, too.
[12]:
n_dem_pnts = demand_points.shape[0]
n_fac_pnts = facility_points.shape[0]
[13]:
process = lambda df: as_gdf(df).sort_values(by=["NAME"]).reset_index(drop=True)
as_gdf = lambda df: geopandas.GeoDataFrame(df, geometry=pnts(df))
pnts = lambda df: geopandas.points_from_xy(df.long, df.lat)
[14]:
facility_points = process(facility_points)
demand_points = process(demand_points)
Reproject the input spatial data.
[15]:
for _df in [facility_points, demand_points, study_area]:
_df.set_crs("EPSG:4326", inplace=True)
_df.to_crs("EPSG:7131", inplace=True)
Here the rest of parameters are set.
ai
is the demand weight and in this case we model as population in year 2000 of this tract.
[16]:
ai = demand_points["POP2000"].to_numpy()
# maximum service radius (in meters)
SERVICE_RADIUS = 5000
# number of candidate facilities in optimal solution
P_FACILITIES = 4
Below, the method is used to plot the results of the four models that we will prepare to solve the problem.
[17]:
dv_colors_arr = [
"darkcyan",
"mediumseagreen",
"saddlebrown",
"darkslategray",
"lightskyblue",
"thistle",
"lavender",
"darkgoldenrod",
"peachpuff",
"coral",
"mediumvioletred",
"blueviolet",
"fuchsia",
"cyan",
"limegreen",
"mediumorchid",
]
dv_colors = {f"y{i}": dv_colors_arr[i] for i in range(len(dv_colors_arr))}
dv_colors
[17]:
{'y0': 'darkcyan',
'y1': 'mediumseagreen',
'y2': 'saddlebrown',
'y3': 'darkslategray',
'y4': 'lightskyblue',
'y5': 'thistle',
'y6': 'lavender',
'y7': 'darkgoldenrod',
'y8': 'peachpuff',
'y9': 'coral',
'y10': 'mediumvioletred',
'y11': 'blueviolet',
'y12': 'fuchsia',
'y13': 'cyan',
'y14': 'limegreen',
'y15': 'mediumorchid'}
[18]:
def plot_results(model, p, facs, clis=None, ax=None):
"""Visualize optimal solution sets and context."""
if not ax:
multi_plot = False
fig, ax = plt.subplots(figsize=(6, 9))
markersize, markersize_factor = 4, 4
else:
ax.axis("off")
multi_plot = True
markersize, markersize_factor = 2, 2
ax.set_title(model.name, fontsize=15)
# extract facility-client relationships for plotting (except for p-dispersion)
plot_clis = isinstance(clis, geopandas.GeoDataFrame)
if plot_clis:
cli_points = {}
fac_sites = {}
for i, dv in enumerate(model.fac_vars):
if dv.varValue:
dv, predef = facs.loc[i, ["dv", "predefined_loc"]]
fac_sites[dv] = [i, predef]
if plot_clis:
geom = clis.iloc[model.fac2cli[i]]["geometry"]
cli_points[dv] = geom
# study area and legend entries initialization
study_area.plot(ax=ax, alpha=0.5, fc="tan", ec="k", zorder=1)
_patch = Patch(alpha=0.5, fc="tan", ec="k", label="Dissolved Service Areas")
legend_elements = [_patch]
if plot_clis:
# any clients that not asscociated with a facility
if model.name.startswith("mclp"):
c = "k"
if model.n_cli_uncov:
idx = [i for i, v in enumerate(model.cli2fac) if len(v) == 0]
pnt_kws = dict(ax=ax, fc=c, ec=c, marker="s", markersize=7, zorder=2)
clis.iloc[idx].plot(**pnt_kws)
_label = f"Demand sites not covered ($n$={model.n_cli_uncov})"
_mkws = dict(marker="s", markerfacecolor=c, markeredgecolor=c, linewidth=0)
legend_elements.append(mlines.Line2D([], [], ms=3, label=_label, **_mkws))
# all candidate facilities
facs.plot(ax=ax, fc="brown", marker="*", markersize=80, zorder=8)
_label = f"Facility sites ($n$={len(model.fac_vars)})"
_mkws = dict(marker="*", markerfacecolor="brown", markeredgecolor="brown")
legend_elements.append(mlines.Line2D([], [], ms=7, lw=0, label=_label, **_mkws))
# facility-(client) symbology and legend entries
zorder = 4
for fname, (fac, predef) in fac_sites.items():
cset = dv_colors[fname]
if plot_clis:
# clients
geoms = cli_points[fname]
gdf = geopandas.GeoDataFrame(geoms)
gdf.plot(ax=ax, zorder=zorder, ec="k", fc=cset, markersize=100 * markersize)
_label = f"Demand sites covered by {fname}"
_mkws = dict(markerfacecolor=cset, markeredgecolor="k", ms=markersize + 7)
legend_elements.append(
mlines.Line2D([], [], marker="o", lw=0, label=_label, **_mkws)
)
# facilities
ec = "k"
lw = 2
predef_label = "predefined"
if model.name.endswith(predef_label) and predef:
ec = "r"
lw = 3
fname += f" ({predef_label})"
facs.iloc[[fac]].plot(
ax=ax, marker="*", markersize=1000, zorder=9, fc=cset, ec=ec, lw=lw
)
_mkws = dict(markerfacecolor=cset, markeredgecolor=ec, markeredgewidth=lw)
legend_elements.append(
mlines.Line2D([], [], marker="*", ms=20, lw=0, label=fname, **_mkws)
)
# increment zorder up and markersize down for stacked client symbology
zorder += 1
if plot_clis:
markersize -= markersize_factor / p
if not multi_plot:
# legend
kws = dict(loc="upper left", bbox_to_anchor=(1.05, 0.7))
plt.legend(handles=legend_elements, **kws)
LSCP¶
[19]:
from spopt.locate import LSCP
lscp = LSCP.from_cost_matrix(cost_matrix, SERVICE_RADIUS)
lscp = lscp.solve(pulp.GLPK(msg=False))
lscp_objval = lscp.problem.objective.value()
lscp_objval
[19]:
8
Define the decision variable names used for mapping.
[20]:
facility_points["dv"] = lscp.fac_vars
facility_points["dv"] = facility_points["dv"].map(lambda x: x.name.replace("_", ""))
facility_points["predefined_loc"] = 0
[21]:
plot_results(lscp, lscp_objval, facility_points, clis=demand_points)
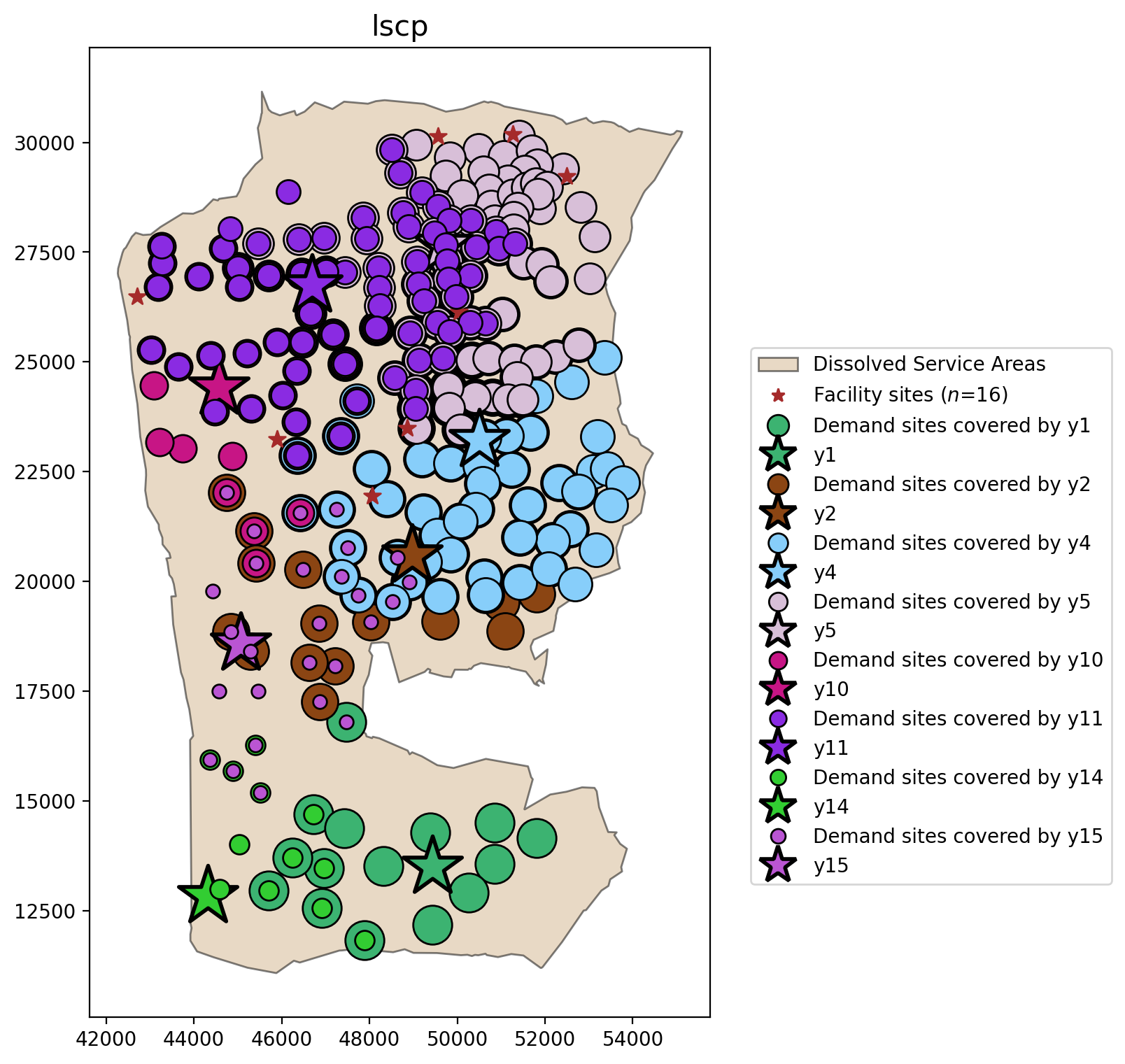
MCLP¶
[22]:
from spopt.locate import MCLP
mclp = MCLP.from_cost_matrix(
cost_matrix, ai, service_radius=SERVICE_RADIUS, p_facilities=P_FACILITIES,
)
mclp = mclp.solve(pulp.GLPK(msg=False))
mclp.problem.objective.value()
[22]:
875247
MCLP Percentage Covered
[23]:
mclp.perc_cov
[23]:
89.75609756097562
[24]:
plot_results(mclp, P_FACILITIES, facility_points, clis=demand_points)
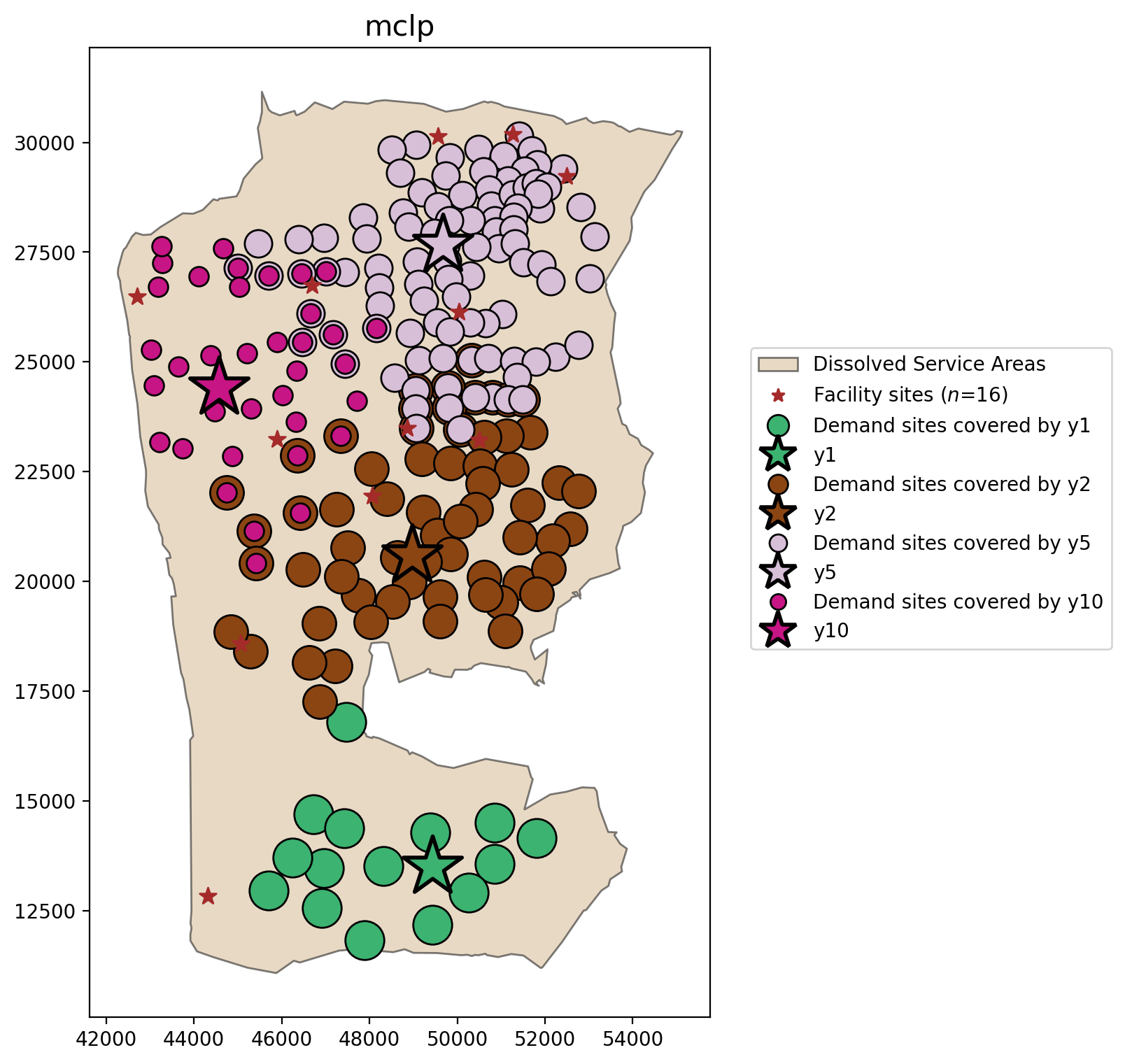
P-Median¶
[25]:
from spopt.locate import PMedian
pmedian = PMedian.from_cost_matrix(cost_matrix, ai, p_facilities=P_FACILITIES)
pmedian = pmedian.solve(pulp.GLPK(msg=False))
pmedian.problem.objective.value()
[25]:
2848268129.7145104
\(p\)-median mean weighted distance
[26]:
pmedian.mean_dist
[26]:
2982.1268579890657
[27]:
plot_results(pmedian, P_FACILITIES, facility_points, clis=demand_points)
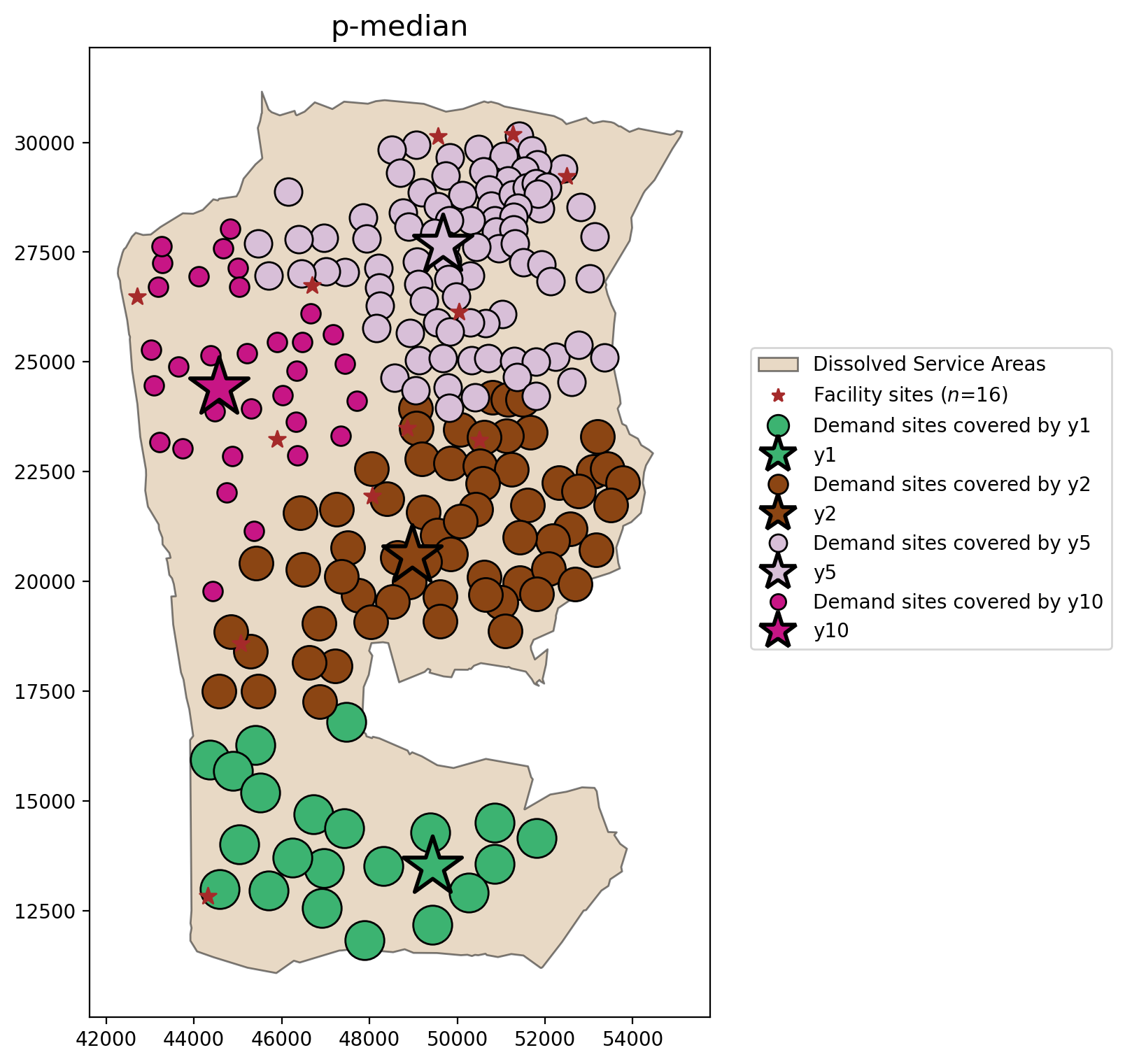
P-Center¶
[28]:
from spopt.locate import PCenter
pcenter = PCenter.from_cost_matrix(cost_matrix, p_facilities=P_FACILITIES)
pcenter = pcenter.solve(pulp.GLPK(msg=False))
pcenter.problem.objective.value()
[28]:
7403.06
[29]:
plot_results(pcenter, P_FACILITIES, facility_points, clis=demand_points)
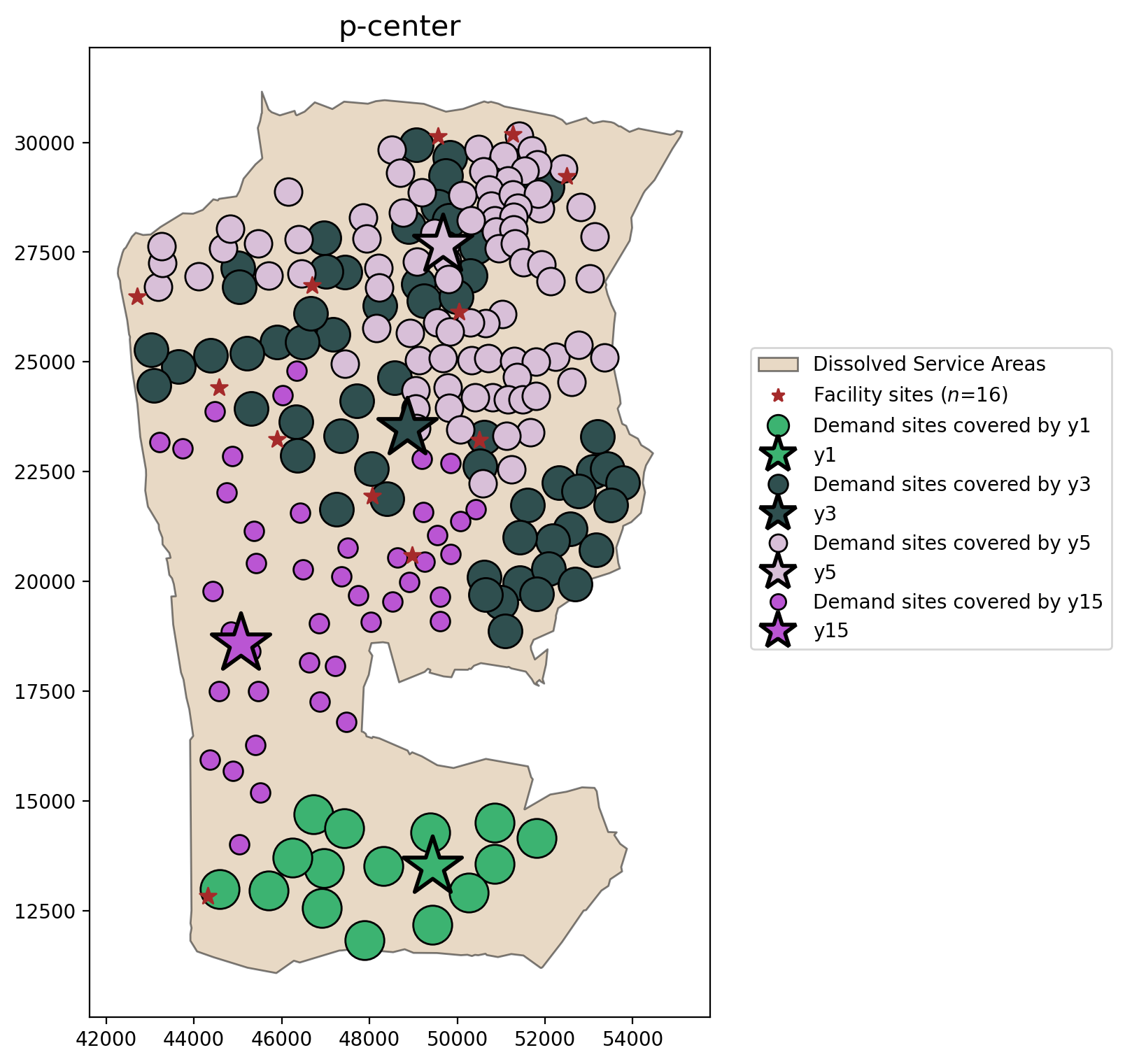
Comparing all models¶
[30]:
fig, axarr = plt.subplots(1, 4, figsize=(20, 10))
fig.subplots_adjust(wspace=-0.01)
for i, m in enumerate([lscp, mclp, pmedian, pcenter]):
_p = m.problem.objective.value() if m.name == "lscp" else P_FACILITIES
plot_results(m, _p, facility_points, clis=demand_points, ax=axarr[i])
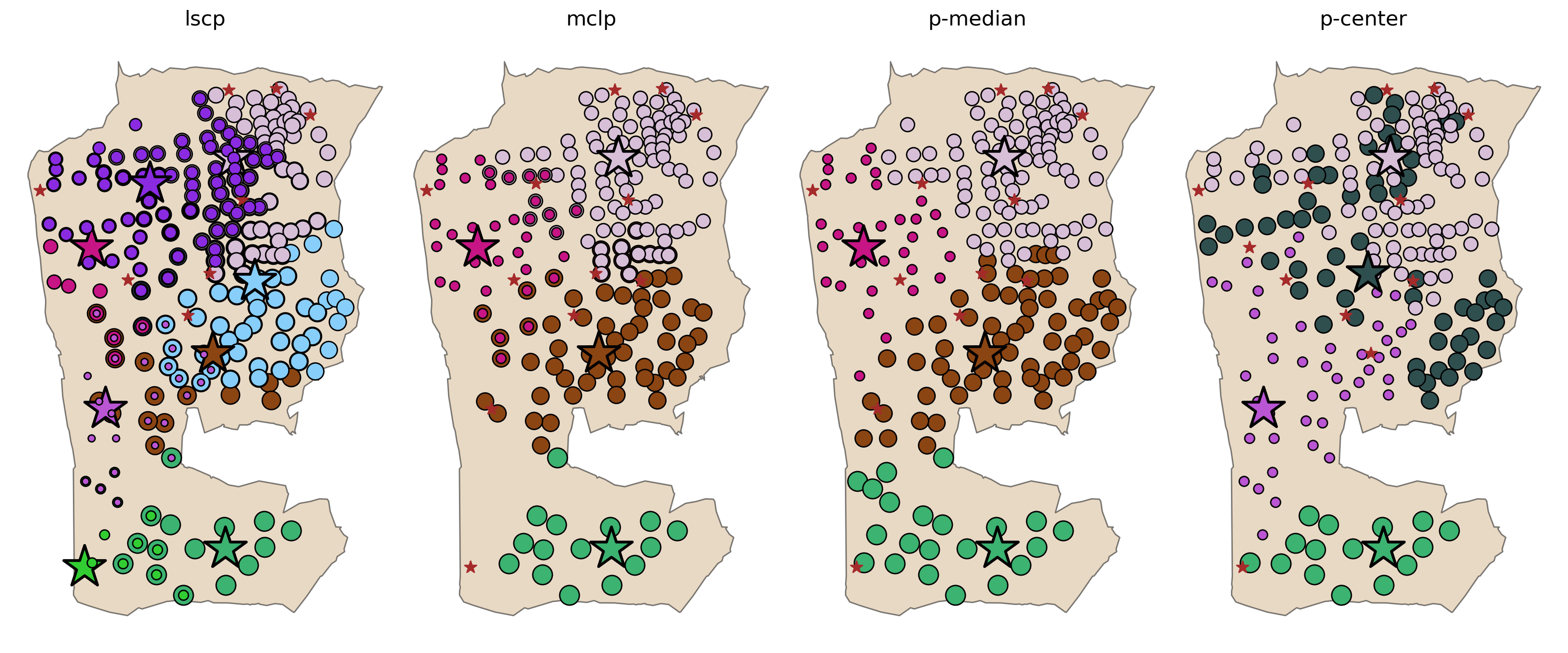
The plot above clearly demonstrates the key features of each location model: * LSCP * Overlapping clusters of clients associated with multiple facilities * All clients covered by at least one facility * MCLP * Overlapping clusters of clients associated with multiple facilities * Potential for complete coverage, but not guranteed * \(p\)-median * Tight and distinct clusters of client-facility relationships * All clients allocated to exactly one facility * \(p\)-center * Loose and overlapping clusters of client-facility relationships * All clients allocated to exactly one facility
Evaluating available solvers¶
For this task we’ll solve the MCLP and \(p\)-center models as examples of solver performance, but first we’ll determine which solvers are installed locally.
[31]:
with warnings.catch_warnings(record=True) as w:
solvers = pulp.listSolvers(onlyAvailable=True)
for _w in w:
print(_w.message)
solvers
GUROBI error:
Failed to set up a license
Error 10009: License expired 2022-08-07
.
[31]:
['GLPK_CMD', 'CPLEX_CMD', 'PULP_CBC_CMD', 'COIN_CMD', 'SCIP_CMD', 'HiGHS_CMD']
Above we can see that it returns a list with different solvers that are available. So, it’s up to the user to choose the best solver that fits the model.
MCLP¶
Let’s get the percentage as a metric to evaluate which solver is the best or improves the model.
[32]:
mclp = MCLP.from_cost_matrix(
cost_matrix, ai, service_radius=SERVICE_RADIUS, p_facilities=P_FACILITIES,
)
[33]:
results = pandas.DataFrame(columns=["Coverage %", "Solve Time (sec.)"], index=solvers)
for solver in solvers:
if solver == "HiGHS_CMD":
# HiGHS doesn't directly support maximization
with warnings.catch_warnings(record=True) as w:
_mclp = mclp.solve(pulp.get_solver(solver, msg=False))
print(w[0].message)
else:
_mclp = mclp.solve(pulp.get_solver(solver, msg=False))
results.loc[solver] = [_mclp.perc_cov, _mclp.problem.solutionTime]
results
HiGHS_CMD does not currently allow maximization, we will minimize the inverse of the objective function.
[33]:
Coverage % | Solve Time (sec.) | |
---|---|---|
GLPK_CMD | 89.756098 | 0.027721 |
CPLEX_CMD | 89.756098 | 0.041584 |
PULP_CBC_CMD | 89.756098 | 0.030322 |
COIN_CMD | 89.756098 | 0.041051 |
SCIP_CMD | 89.756098 | 0.043232 |
HiGHS_CMD | 89.756098 | 0.030545 |
As is expected from such a small model the Coverage %, and thus the obejective values, are equivalent for all solutions across solvers. However, solve times do vary slightly.
P-Center¶
[34]:
pcenter = PCenter.from_cost_matrix(cost_matrix, p_facilities=P_FACILITIES)
[35]:
results = pandas.DataFrame(columns=["MinMax", "Solve Time (sec.)"], index=solvers)
for solver in solvers:
_pcenter = pcenter.solve(pulp.get_solver(solver, msg=False))
results.loc[solver] = [
_pcenter.problem.objective.value(), _pcenter.problem.solutionTime
]
results
[35]:
MinMax | Solve Time (sec.) | |
---|---|---|
GLPK_CMD | 7403.06 | 23.979474 |
CPLEX_CMD | 7403.063811 | 0.739071 |
PULP_CBC_CMD | 7403.0638 | 1.751734 |
COIN_CMD | 7403.0638 | 1.821592 |
SCIP_CMD | 7403.063811 | 6.797391 |
HiGHS_CMD | 7403.063811 | 2.603489 |
Here the MinMax objective values are mostly equivalent, but there are some precision concerns. This is due to the computational complexity of the \(p\)-center problem, which also results in dramaticaly longer run times needed to isolate the optimal solutions (when compared with the MCLP above).