This page was generated from /home/runner/work/esda/esda/docs/notebooks/LOSH.ipynb.
Interactive online version:
Assessing local patterns of spatial heteroskedasticity¶
In the following notebook we review the local spatial heteroskedasticity (LOSH) statistic (\(H_i\)) put forward by Ord and Getis (2012). LOSH is meant as an accompainment to the local statistics that analyze the mean level of a spatial process. LOSH focuses on analyzing the variance of the spatial process.
As outlined by Ord and Getis, consider a 10 x 10 grid of property values. Within this grid, there is a central high-rent district (identified by cells that are 1) and a surrounding lower-value area (identified by cells that are 0. We can visualize this spatial arrangement as:
0 |
0 |
0 |
0 |
0 |
0 |
0 |
0 |
0 |
0 |
0 |
0 |
0 |
0 |
0 |
0 |
0 |
0 |
0 |
0 |
0 |
0 |
0 |
0 |
0 |
0 |
0 |
0 |
0 |
0 |
0 |
0 |
0 |
1 |
1 |
1 |
1 |
0 |
0 |
0 |
0 |
0 |
0 |
1 |
1 |
1 |
1 |
0 |
0 |
0 |
0 |
0 |
0 |
1 |
1 |
1 |
1 |
0 |
0 |
0 |
0 |
0 |
0 |
1 |
1 |
1 |
1 |
0 |
0 |
0 |
0 |
0 |
0 |
0 |
0 |
0 |
0 |
0 |
0 |
0 |
0 |
0 |
0 |
0 |
0 |
0 |
0 |
0 |
0 |
0 |
0 |
0 |
0 |
0 |
0 |
0 |
0 |
0 |
0 |
0 |
While we can see that the inner core of the high-rent district have similar values to their neigbhors (i.e. a cells of 1 surrounded by other cells of 1), this is less true when we consider the outer rim of the high-rent district. This rim represents a possible transition region between the stable inner high-rent area and the stable outer lower value areas. Ord and Getis seek to use the LOSH statistic (\(H_i\)) to identify this transitional region between the two areas.
Understanding the LOSH statistic¶
Ord and Getis begin by defining a local mean, \(\bar{x}_i (d)\), as a re-scaled form of their \(G^*_i\) statistic. This local mean is:
Eq. 1
With a local mean defined, we can understand local residuals as the value of a local unit minus the local mean, or:
Eq. 2
These local residuals may be incorporated into Eq. 1 to form a final local dispersion statistic called \(H_i\):
Eq. 3
Note the addition of a new variable, \(a\). This handles the interpretation of the residuals. As explaiend by Ord and Getis:
When a = 1, we have an absolute deviations measure, Hi 1, and when a = 2 a variance measure, Hi2. Clearly, other choices are possible, along with various robust forms to avoid outliers. In order to produce a standard measure, we should divide by the mean absolute deviation or variance for the whole data set.
The default settings of the losh()
function set \(a=2\).
Interpreting the LOSH statistic¶
Ord and Getis suggest that the \(H_i\) statistic may benefit from interpretation with the \(G^*_i\) statistic. The logic of this combined interpretation is that a the \(G^*_i\) value speaks to the local mean of the phenomenon of interest, whereas the \(H_i\) speaks to the local heterogeneity. Ord and Getis provide the following table as a simplified guide for interpretation:
Mean:nbsphinx-math:variance |
High \(H_i\) |
Low \(H_i\) |
---|---|---|
Large \(|G^*_i|\) |
A hot spot with heterogeneous local conditions |
A hot spot with similar surrounding areas; the map would indicate whether the affected region is larger than the single ‘cell’ |
Small \(|G^*_i|\) |
Heterogeneous local conditions but at a low average level (an unlikely event) |
Homogeneous local conditions and a low average level |
Inference on the LOSH statistic¶
The current inference in the PySAL implementation of the LOSH statistic uses a \(\chi^2\) distribution. For each unit, we calculate a Z-score as:
with \(2/V_i\) degrees of freedom. \(V_i\) is the local variance of the unit, calculated as:
It is worthwhile to note that alternative methods for inference have been proposed in Xu et al (2014). While these methods are not yet implemented in PySAL, they are available in the R
spdep
package as LOSH.mc
. The PySAL function is comparable to the spdep
LOSH
and LOSH.cs
functions.
Applying the LOSH statistic on a dataset¶
As a point of comparison, we now demonstrate the PySAL losh
function on the Boston Housing Dataset, which is also used as the docexample in R
spdep
LOSH.cs
. We are interested in the variable NOX
, which is the ‘…vector of nitric oxides concentration (parts per 10 million) per town’.
We first load the Bostonhsg
example dataset from libpysal
:
[1]:
import geopandas
import libpysal
boston = libpysal.examples.load_example("Bostonhsg")
boston_ds = geopandas.read_file(boston.get_path("boston.shp"))
Then we construct a Queen weight structure:
[2]:
w = libpysal.weights.Queen.from_dataframe(boston_ds, use_index=False)
We can now import the losh()
function. Note that it is in the form of a scikit-learn type estimator, so we pass both a series of arguments and then call .fit()
.
[3]:
from esda.losh import LOSH
ls = LOSH(connectivity=w, inference="chi-square").fit(boston_ds["NOX"])
We can now examine the LOSH (\(H_i\)) values and their significance.
[4]:
ls.Hi[0:10]
[4]:
array([0.19690679, 0.51765774, 0.80382881, 0.80854441, 0.530667 ,
0.525579 , 0.83291425, 0.84215733, 0.48875154, 0.41955327])
[5]:
ls.pval[0:10]
[5]:
array([0.86292242, 0.61157688, 0.45697742, 0.34426167, 0.57934554,
0.55430556, 0.4135546 , 0.40999792, 0.54025022, 0.57801529])
If we want to map the LOSH (\(H_i\)) values, we need to add them back to the boston_ds
dataframe.
[6]:
boston_ds["Hi"] = ls.Hi
boston_ds["Hi_pval"] = ls.pval
We can now map the LOSH (\(H_i\)) values. Note that we choose a Quantile break scheme here for visualization purposes.
[7]:
import matplotlib.pyplot as plt
fig, ax = plt.subplots(figsize=(12, 10), subplot_kw={"aspect": "equal"})
boston_ds.plot(column="Hi", scheme="Quantiles", k=5, cmap="GnBu", legend=True, ax=ax)
[7]:
<Axes: >
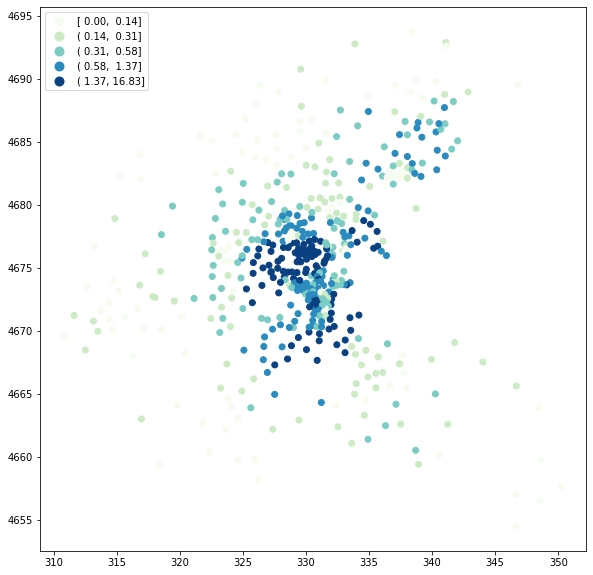
We can also examine significance of the values. We use cutoffs of 0.01, 0.05, 0.10, and above 0.10.
[8]:
fig, ax = plt.subplots(figsize=(12, 10), subplot_kw={"aspect": "equal"})
boston_ds.plot(
column="Hi_pval",
cmap="RdBu",
legend=True,
ax=ax,
scheme="user_defined",
classification_kwds={"bins": [0.01, 0.05, 0.10, 1]},
)
[8]:
<Axes: >
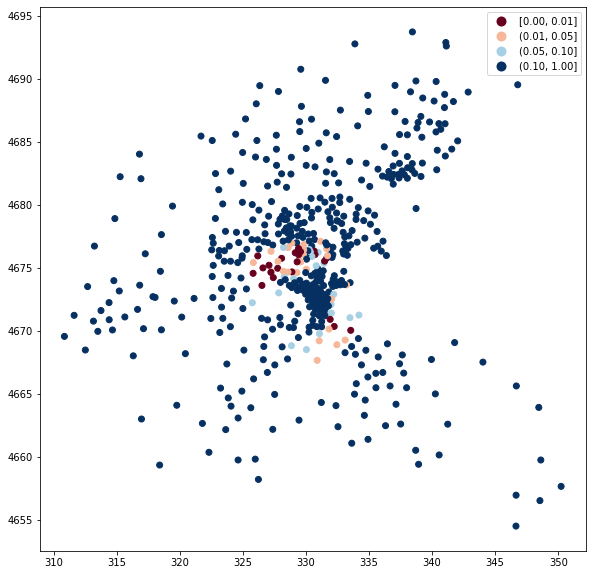